I was recently creeping through a clearing of downed trees in a wooded Brooklyn park with my iPhone in hand. Birds were singing everywhere, but through the din, I was recording a peculiar song: It was almost certainly the slurred, metallic whistle of a Bicknell’s thrush. Though a plain-looking, brown-speckled bird, this rare thrush is a prime target of New York City’s birdwatchers — but its identification poses a challenge. Unless you’re holding it in your hand, you can’t reliably identify it based on its appearance alone, and its song differs only slightly from its doppelganger, the more common grey-cheeked thrush.
I left the copse with only a muddied recording of the experience, one littered with background noise and the chirping of other birds. But when I uploaded the file to the Merlin Bird ID app’s new Sound ID feature, it correctly named every bird in the recording, including cardinals and warblers, and it could discern between the faint whistle of the Bicknell’s and grey-cheeked thrushes that were both on the recording.
Plenty of apps attempt to identify birds from images and sounds, with varying levels of success — one app I was asked to review called every recording a northern mockingbird, a bird that mimics other birds. But birders and citizen scientists have long relied on the Cornell Lab of Ornithology’s Merlin Bird ID as a go-to for identification assistance on bird photos. When I found out that they’d expanded their services to birdsong, I was quick to try it out and eager to learn more about what’s behind machine learning-powered sound identification.
Experienced birders can readily identify birds by their unique songs, but doing so can be difficult and takes time and experience. Such is the purpose of Merlin Bird ID — to help those still trying to figure things out. “The cool thing about Merlin is that it’s a non-judgmental companion who can tell you that you’re hearing a song sparrow for the 300th time, and will tell you as happily as it did the first time,” said Drew Weber, the Merlin Bird ID project coordinator.
I took the app for a dedicated test drive this past weekend in Brooklyn’s Prospect Park to ensure that its success on the previous recording wasn’t a fluke. Though the city’s location and ecology make it a prime birdwatching destination during the spring and fall, only a few songbirds remain in the parks during the summer, so the app would have the advantage of having mainly common birds to identify.
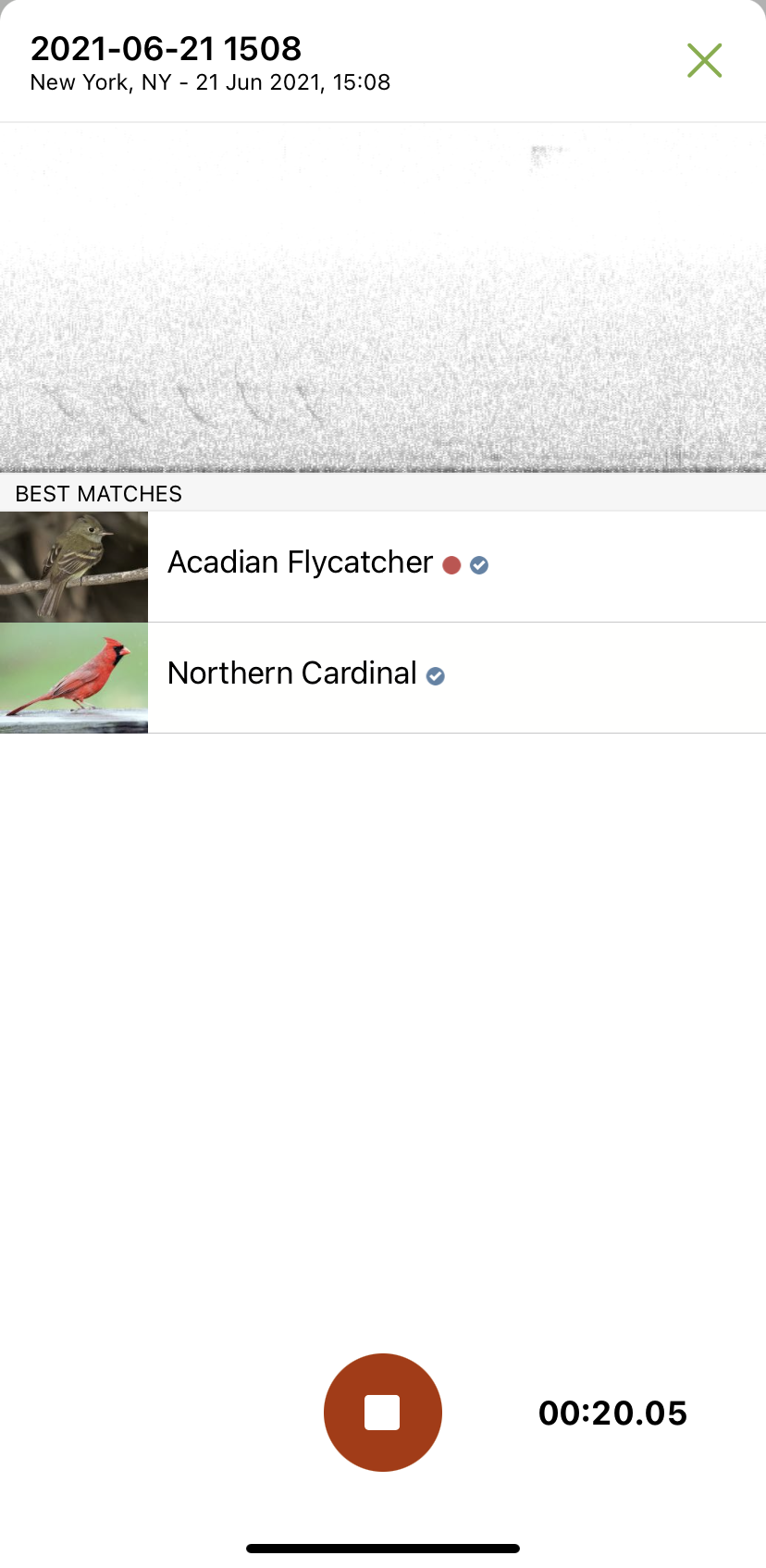
I stopped at a tree by the park’s noisy southwest entrance, where a Baltimore oriole was singing from a pine tree. I booted up the Sound ID feature, hit record, and held my phone over my head. The app showed me a spectrogram — a graph of the frequencies it was recording over time — and immediately suggested “American robin;” indeed, a robin had started singing behind me. I tried again, and this time, a house sparrow started cheeping. The app showed me a house sparrow’s photo. I tried one final time, and right as the oriole sang, a chimney swift made its tinkling chitter from above; the app responded that it had once again ignored the oriole in favour of correctly identifying something else. I suppose this demonstrated the nimbleness with which the app could offer an identification, but I was frustrated that it failed to identify the oriole — a common bird — in this easy setting.
As I hiked into the park woods, I kept the app open and recording for any other birds I might encounter. It successfully identified a northern cardinal’s “pew-pew-pew” song, though when the cardinal started making a high-pitched chip note, the app hilariously suggested that I was now listening to an osprey, a huge, fish-eating hawk. The loud, high-pitched “seeee” notes of cedar waxwings appeared crisply on the spectrogram, though the sound went unidentified, and instead an image of a warbling vireo popped up as one began singing in the distance (a song I’ve heard described as “a drunk person trying to make a point”).
Merlin’s Sound ID won me over, though; I barely heard a distant pair of notes, and immediately the app suggested Acadian flycatcher, a bird of southeastern forests that’s uncommon in New York but occasionally nests in Prospect Park. I walked deeper into the woods, since the app heard the bird better than I had. Sure enough, I was soon standing beneath a tree from which the small, greenish bird sang an emphatic “pwee-tseet!”
Merlin Bird ID is more than just a sound identification app, though; it’s the result of tens of thousands of bird watchers and citizen scientists submitting over a million avian audio recordings to Cornell’s Macaulay Library through the eBird app in just the past few years. Given the volume of data, Weber and Macaulay Library research engineer Grant Van Horn, plus other members of the Cornell Lab of Ornithology, wondered last summer what it might take to create a birdsong identifying feature of the Merlin Bird ID app.
Sound identification is, in fact, an image recognition problem, Van Horn explained. Caltech and Cornell Tech engineers had already put together an image recognition neural network toolkit for birds using photos from the Macaulay Library to create the Merlin Photo ID feature. Sound ID converts audio into spectrogram images, processes them, and then traditional computer vision tools compares these spectrograms to spectrograms of existing bird recordings.
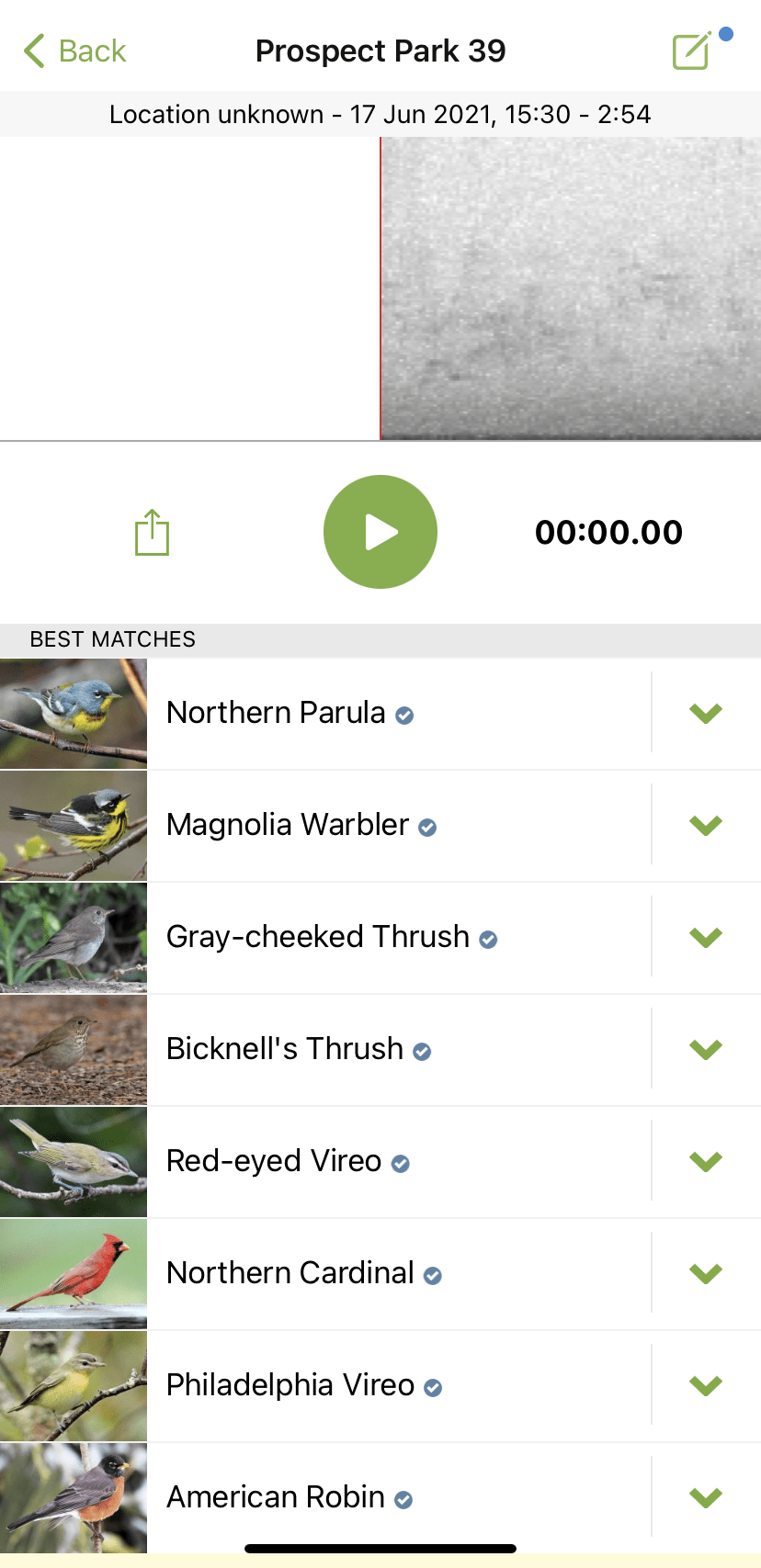
Crucial to the identification process is a robust training dataset — which required the help of citizen scientists, explained Weber. Like my Bicknell’s thrush recording, the Macaulay Library’s recordings often have many species singing in the background. A team of volunteer annotators went through the training set of spectrograms from over 400 North American bird species, drawing boxes around and labelling each individual species’ sounds. The result was a dataset with around 250,000 annotations, each box corresponding to only one species. Users of the app either upload a file or record the birds live, and the app will return every bird it hears for every three seconds of audio. The team also trained the algorithm on a wide variety of background noises, including Google’s expansive AudioSet dataset, so that the app was aware of what non-birds sound like.
There are other high-quality birdsong identifying apps — in fact, the Cornell Lab of Ornithology, together with the Chemnitz University of Technology, also runs the BirdNET Sound ID app. However, those apps have slightly different purposes: BirdNET serves mainly as a research tool for scientists, while Merlin is instead a citizen science-powered bird identification app that also includes photo and Q+A identification, a built-in field guide, and data from the eBird citizen science database of bird sightings, sounds, and images. Data from eBird also helps power the Merlin Sound and Photo ID features; they rely on citizen scientist records of nearby birds in order to make more accurate recommendations.
There’s plenty of room for Merlin’s Sound ID to grow. There are 10,000 birds, and the app only recognises around 400 of them right now. Short chirps pose a challenge, since they can sound extremely similar between species, while the app might mistake certain low-frequency songs for background noise. But as the dataset improves, so too will the machine learning algorithm and the app’s capabilities.
Van Horn was excited about the potential for the dataset and machine learning model. He plans to use the model in other areas of the Cornell Lab of Ornithology, such as on bird cams with a steady stream of audio. Weber said that perhaps they can use the model to tell what birds are flying over cities during the peak of bird migration, Perhaps they can use the model to recognise videos of birds, as well. Van Horn also told me that he thinks about bias and other ethical issues of machine learning, and pointed out that this algorithm is intended solely for wildlife, was created using only data that users consented to giving Cornell via eBird, and runs on the user’s phone without sending data back to Cornell.
The fact that there’s a sound identification feature in one of the most popular bird-identifying apps will be welcome news to plenty of birders, and after trying it out, I can confidently say that it works decently. Experienced birders may still find that their ears are a little more accurate than the app, but, at least for me, the tool was a welcome addition to my bird-identifying toolkit.